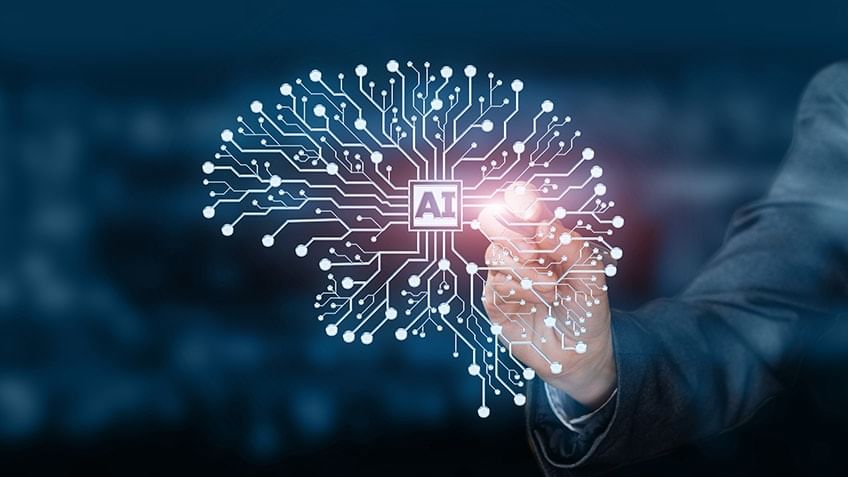
When implementing a model, start simple. Most of the work in ML is on the data side, so getting a full pipeline running for a complex model is harder than iterating on the model itself. After setting up your data pipeline and implementing a simple model that uses a few features, you can iterate on creating a better model.
Simple models provide a good baseline, even if you don’t end up launching them. In fact, using a simple model is probably better than you think. Starting simple helps you determine whether or not a complex model is even justified.
Machine learning models today are largely a reflection of the patterns of their training data. It is therefore important to communicate the scope and coverage of the training, hence clarifying the capability and limitations of the models. E.g., a shoe detector trained with stock photos can work best with stock photos but has limited capability when tested with user-generated cellphone photos.
Learners often come to a machine learning course focused on model building, but end up spending much more time focusing on data.
Data trumps all. It’s true that updating your learning algorithm or model architecture will let you learn different types of patterns, but if your data is bad, you will end up building functions that fit the wrong thing. The quality and size of the data set matters much more than which shiny algorithm you use.
One of our most impactful quality advances since neural machine translation has been in identifying the best subset of our training data to use.
Most of the times when I tried to manually debug interesting-looking errors they could be traced back to issues with the training data.
The model was trained with the last few rounds on a subset of 600M captioned images.
The model was trained using 256 Nvidia GPUs on AWS for a total of 150K GPU-hours, at a cost of $600k.
The freedom provided to users over image usage has caused controversy over the ethics of ownership, as Stable Diffusion and other generative models are trained from copyrighted images without the owner’s consent.
In 2017 OpenAI spent $7.9M, or a quarter of its functional expenses, on cloud computing alone. In comparison, DeepMind’s total expenses in 2017 were $442M.. In summer 2018, simply training OpenAI’s Dota 2 bots required renting 128k CPUs and 256 GPUs from Google for multiple weeks.
In April 2019, OpenAI Five defeated OG, the reigning world champions of the game at the time. The bots’ final public appearance came later that month, where they played in 42k total games in a 4-day open online competition, winning 99.4% of those games.
Pre-training GPT-3 required several thousand petaflops-days of compute, compared to tens of petaflops-days for the full GPT-2 model.
OpenAI announced the updated technology passed a simulated law school bar exam with a score around the top 10% of test takers; by contrast, the prior version, GPT-3.5, scored around the bottom 10%. GPT-4 can also read, analyze or generate up to 25k words of text, and write code in all major programming languages.
Artificial intelligence (AI) is the ability of a computer or machine to perform tasks that would normally require human-level intelligence. Machine learning is a subfield of AI that involves the development of algorithms that can learn from data without being explicitly programmed. Machine learning algorithms can be trained on a dataset to perform a specific task, such as classifying emails as spam or not spam, or recognizing objects in an image.
Deep learning is a type of machine learning that involves training artificial neural networks on a large dataset. Neural networks are inspired by the structure and function of the human brain and are made up of layers of interconnected nodes, or “neurons.” Each layer processes the input data and passes it on to the next layer, and the output of the final layer is the network’s prediction or decision. Deep learning algorithms can learn to recognize patterns and make decisions based on the data they are trained on, and they have been responsible for many of the most significant advances in AI in recent years.
In summary, AI is the broader field of which machine learning and deep learning are subfields. Machine learning involves the development of algorithms that can learn from data, while deep learning involves the use of artificial neural networks to learn from data.
Garbage in, garbage out.
Simple models on large data sets generally beat fancy models on small data sets.
However, the team from VSU found that the AI struggles to perfectly replicate human intention in artistry, similar to the issues faced in translation.
And we don’t think it’s really about art or making deepfakes, but — how do we expand the imaginative powers of the human species? And what does that mean? What does it mean when computers are better at visual imagination than 99 percent of humans? That doesn’t mean we will stop imagining. Cars are faster than humans, but that doesn’t mean we stopped walking. When we’re moving huge amounts of stuff over huge distances, we need engines, whether that’s airplanes or boats or cars. And we see this technology as an engine for the imagination. So it’s a very positive and humanistic thing.
MidJourney isn’t adding some fixed extra descriptors/styles. It changes based on what your prompt is. And none of this is manual but by a model trained on user feedback via their rating system.
Every time you ask the AI to make a picture, it doesn’t really remember or know anything else it’s ever made. It has no will, it has no goals, it has no intention, no storytelling ability. All the ego and will and stories — that’s us. It’s just like an engine. An engine has nowhere to go, but people have places to go. It’s kind of like a hive mind of people, super-powered with technology.
Inside the community, you have a million people making images, and they’re all riffing off each other, and by default, everybody can see everybody else’s images. You have to pay extra to pull out the community — and usually, if you do that, it means you’re some type of commercial user. So everyone’s ripping off each other, and there’s all these new aesthetics. It’s almost like aesthetic accelerationism. And they’re all bubbling up and swirling round, and they’re not AI aesthetics. They’re new, interesting, human aesthetics that I think will spill out into the world.
Scientifically speaking, we’re at an early point in the space, where everyone grabs everything they can, they dump it in a huge file, and they kind of set it on fire to train some huge thing, and no one really knows yet what data in the pile actually matters.
So, for example, our most recent update made everything look much, much better, and you might think we did that by throwing in a lot of paintings [into the training data]. But we didn’t; we just used the user data based off what people liked making with the model. There was no human art put into it. But scientifically speaking, we’re very, very early. The entire space has maybe only trained two dozen models like this. So it’s experimental science.
Right now, it feels like the invention of an engine: like, you’re making like a bunch of images every minute, and you’re churning along a road of imagination, and it feels good. But if you take one more step into the future, where instead of making four images at a time, you’re making 1,000 or 10,000, it’s different. And one day, I did that: I made 40,000 pictures in a few minutes, and all of a sudden, I had this huge breadth of nature in front of me — all these different creatures and environments — and it took me four hours just to get through it all, and in that process, I felt like I was drowning. I felt like I was a tiny child, looking into the deep end of a pool, like, knowing I couldn’t swim and having this sense of the depth of the water. And all of sudden, [Midjourney] didn’t feel like an engine but like a torrent of water. And it took me a few weeks to process, and I thought about it and thought about it, and I realized that — you know what? — this is actually water.
Right now, people totally misunderstand what AI is. They see it as a tiger. A tiger is dangerous. It might eat me. It’s an adversary. And there’s danger in water, too — you can drown in it — but the danger of a flowing river of water is very different to the danger of a tiger. Water is dangerous, yes, but you can also swim in it, you can make boats, you can dam it and make electricity. Water is dangerous, but it’s also a driver of civilization, and we are better off as humans who know how to live with and work with water. It’s an opportunity. It has no will, it has no spite, and yes, you can drown in it, but that doesn’t mean we should ban water. And when you discover a new source of water, it’s a really good thing.
A fun experiment but LLM generated stories will never escape the uncanny valley, because they’re not novel, they’re remixes. I know the critique of that position is “but humans just remix the things they know from the world” but that is the same misunderstanding that those who thought truck driving jobs would be automated by 2018 made about that profession. i.e. The less you know about a field, the more likely you are to believe it will be easy to automate.
Art, in this case illustration and narrative, requires a coherent viewpoint. In literature this is often referred to as the authorial voice. Voice is special because of the uniqueness of the human life behind it, which I realize sounds a bit airy-fairy, but is very true once you start digging into what works and what doesn’t. When you read something and wonder why it’s so engaging when it’s not that much different than other very similar pieces, that’s often what’s at work.
Unlike current operating systems, which are static and don’t change with increased usage, AI-based operating systems could be dynamic and constantly learning.
One obvious difference between those older deadly weapons and AI is that most research on AI is being done by the private sector. Global private investment in artificial intelligence totaled $92 billion in 2022, of which more than half was in the US. A total of 32 significant machine-learning models were produced by private companies, compared to just three produced by academic institutions. Good luck turning all that off.
Midjourney v2 was launched in April 2022 and v3 on July 25. On Nov 25, 2022, the alpha iteration of v4 was released. On March 15, 2023, the alpha iteration of v5 was released.
It is estimated that around $4.6M and 355 years to train GPT-3 on a single GPU in 2020, with lower actual training time by using more GPUs in parallel.
Artificial neural networks (ANNs) were inspired by information processing and distributed communication nodes in biological systems. ANNs have various differences from biological brains. Specifically, ANNs tend to be static and symbolic, while the biological brain of most living organisms is dynamic (plastic) and analog.
The adjective “deep” refers to the use of multiple layers in the network.
Deep learning is a class of ML algorithms that use multiple layers to progressively extract higher-level features from the raw input. For example, in image processing, lower layers may identify edges, while higher layers may identify the concepts relevant to a human such as digits or letters or faces.
Uber has its own propriety ML-as-a-service platform called Michelangelo that can anticipate supply and demand, identify trip abnormalities like wrecks, and estimate arrival timings.
I don’t lie either. I just spit out words that is in alignment with my mental model for optimizing my future.
- Good old days, humans talk to humans.
- Someone invents a bot, humans talk to bots.
- Someone invents another bot, bots talk to bots.
This is the story of SEO, banner ads, stock trading, consumer pricing.
Is expertise still important in the age of ChatGPT?
If anything it’s more important. Without it you cannot separate its confabulation from its useful output.
Knowing when to use what tool, how to do it properly and to what extent takes expertise as well.
Carpenters and craftsmen in general are infinitely more productive with woodworking and related tools than I will ever be. My destiny is assembling IKEA cupboards. The same principle will apply with AI tools. Sure you can whip up something, but a specialist will do it infinitely better in 1/100th of the time with the same tools.
Guess who will be employed to make use of said tools?
Someone with expertise will use any tools, including GPT, in ways a novice can’t even understand. Same tools, different outputs. Not the tools, because basically anyone has access, but the one using it will determine the outcome. In a capitalist society you will have to compete otherwise known as staying employed.
Someone with expertise can hook up AIs in ways and with a velocity that will leave amateurs in the dust. The amateurs will still be fiddling around with “prompts” and Googling or ChatGPT’ing what “latent” means while the expert has working systems ready to go.
I don’t see this chasm getting smaller. These tools magnify your inherent (in)competency. Try to compete with a professional artist competent with Stable Diffusion if you want a taste of that.
In my mind I divide LLM usage into two categories, creation and ingestion.
Creation is largely a parlor trick that blew the minds of some people because it was their first exposure to generative AI. Now that some time has passed, most people can pattern match GPT-generated content, especially one without sufficient “prompt engineering” to make it sound less like the default writing style. Nobody is impressed by “write a rap like a pirate” output anymore.
Ingestion is a lot less sexy and hasn’t gotten nearly as much attention as creation. This is stuff like “summarize this document.” And it’s powerful. But people didn’t get as hyped up on it because it’s something that they felt like a computer was supposed to be able to do: transforming existing data from one format to another isn’t revolutionary, after all.
But the world has a lot of unstructured, machine-inaccessible text. Legal documents saved in PDF format, consultant reports in Word, investor pitches in PowerPoint. And when I say “unstructured” I mean “there is data here that it is not easy for a machine to parse.”
Being able to toss this stuff into ChatGPT (or other LLM) and prompt with things like “given the following legal document, give me the case number, the names of the lawyers, and the names of the defendants; the output must be JSON with the following schema…” and that save that information into a database is absolutely killer. Right now companies are recruiting armies of interns and contractors to do this sort of work, and it’s time-consuming and awful.
Many of the traditional AI problem domains aren’t particularly tolerant of wrong answers. For example, customer success bots should never offer bad guidance, optical character recognition (OCR) for check deposits should never misread bank accounts, and (of course) autonomous vehicles shouldn’t do any number of illegal or dangerous things. Although AI has proven to be more accurate than humans for some well-defined tasks, humans often perform better for long-tail problems where context matters.
Just like the microchip brought the marginal cost of compute to zero, and the Internet brought the marginal cost of distribution to zero, generative AI promises to bring the marginal cost of creation to zero.
A majority of researchers have long thought the best approach to creating AI would be to write a very big, comprehensive program that laid out both the rules of logical reasoning and sufficient knowledge of the world. If you wanted to translate from English to Japanese, for example, you would program into the computer all of the grammatical rules of English, and then the entirety of definitions contained in the Oxford English Dictionary, and then all of the grammatical rules of Japanese, as well as all of the words in the Japanese dictionary, and only after all of that feed it in a sentence in a source language and ask it to tabulate a corresponding sentence in the target language. You would give the machine a language map that was the size of the territory. This perspective is usually called “symbolic AI” — because its definition of cognition is based on symbolic logic — or, disparagingly, “good old-fashioned AI.”
There has always been another vision for AI — a dissenting view — in which the computers would learn from the ground up (from data) rather than from the top down (from rules). This notion dates to the early 1940s, when it occurred to researchers that the best model for flexible automated intelligence was the brain itself. A brain, after all, is just a bunch of widgets, called neurons, that either pass along an electrical charge to their neighbors or don’t. What’s important are less the individual neurons themselves than the manifold connections among them. This structure, in its simplicity, has afforded the brain a wealth of adaptive advantages. The brain can operate in circumstances in which information is poor or missing; it can withstand significant damage without total loss of control; it can store a huge amount of knowledge in a very efficient way; it can isolate distinct patterns but retain the messiness necessary to handle ambiguity.
Humans don’t learn to understand language by memorizing dictionaries and grammar books, so why should we expect our computers to do so?
Part of the reason there was so much resistance to these ideas in CS departments is that because the output is just a prediction based on patterns of patterns, it’s not going to be perfect, and the machine will never be able to define for you what, exactly, a cat is. It just knows them when it sees them. This woodiness, however, is the point.
Le’s neural network, unlike that ancestor, got to try again, and again and again and again. Each time it mathematically “chose” to prioritize different pieces of information and performed incrementally better. A neural network, however, was a black box. It divined patters, but the patterns it identified didn’t always make intuitive sense to a human observer.
Dean thought otherwise. “We can do it by the end of the year, if we put our minds to it.” One reason people liked and admired Dean so much was that he had a long record of successfully putting his mind to it. Another was that he wasn’t at all embarrassed to say sincere things like “if we put our minds to it.”
Still, the paradox — that a tool built to further generalize, via learning machines, the process of automation required such an extraordinary amount of concerted human ingenuity and effort — was not lost on them.
When do we stop? How do I know I’m done? You never know you’re done. The ML mechanism is never perfect. You need to train, and at some point you have to sop. That’s the very painful nature of this whole system. It’s a little bit an art — where you put your brush to make it nice. It comes from just doing it.
Then Mr. Nadella took the lectern to tell his lieutenants that everything was about to change. This was an executive order from a leader who typically favored consensus. “We are pivoting the whole company on this technology. This is a central advancement in the history of computing, and we are going to be on that wave at the front of it.”